Marketing (or anything, really) as problem solving
Some practical approaches to solving real-world business problems
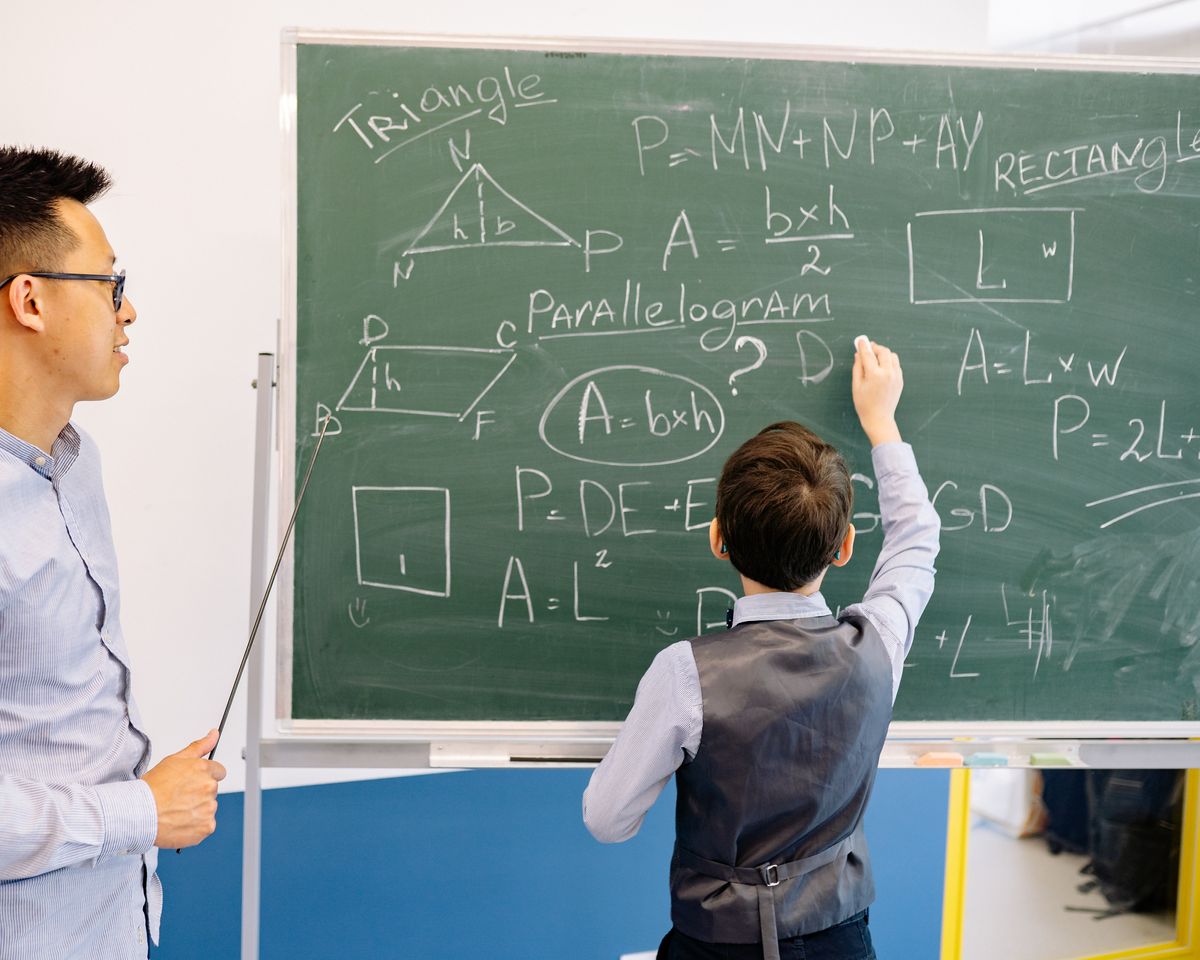
How should we really think about marketing? Think deeply enough and it becomes almost infinitely complex. Yet stripped to its basics it feels trivially simple.
Over the past decade, we’ve become accustomed to goals — conversions, awareness, market share to name a few. But without thorough comprehension of how to actually achieve these goals, many efforts flop.
So here's my proposal: we should stop bucketing ourselves as creatives, growth experts or channel specialists. Instead, we should think of ourselves as problem solvers. This way, we can treat each company, campaign and strategy on their own merits. This still leaves space to hone skills within different specialisms, but fundamentally — we are solving problems.
Contrary to some beliefs, marketing is hard. It intersects consumer psychology, finance, design, product and data. Crucially, we're dealing with people — and it's onerous to know exactly what's going on inside their heads.
And at its best, good marketing can be the primary generative function for a business (assuming you have a good product, of course). This may have accidentally lead to the rise of the growth marketing professional — almost a way of showing in a more quantitative way that marketing can and does grow companies.
It’s worth noting the guidelines I provide in this essay are, ostensibly, relevant almost all other business functions. Perhaps other life challenges too. But a large part of developing competence in a role is to recognize the uniqueness of your business problem in the right context.
This doesn’t mean ripping out playbooks, frameworks and heuristics entirely — rather learning to apply them to relevant problems. Not merely retrofitting.
So let's dive into what good problem solving looks like.
Step 1: Formulate The Problem
Subgoal #1: Diagnosis
Subgoal #2: Converge to relevance realisation
Subgoal #3: State the well-formulated problem(s)
Understanding which problem to solve and where to direct your strategy begins with knowledge of your initial state. Stated clearly: you must discern how to properly formulate your problem. Everything everything else rests upon the accurate formulation — after all, can you solve a problem you don’t precisely understand?
For the sake of the remainder of this essay, I’ll use the hypothetical problem of Company X — a consumer SaaS business seeking to double its sales of the next 12 months.
This is deliberately vague problem statement. But it’s the type of arbitrary, open problem many teams face (or have thrust upon them by management).
At this stage it’s worth highlighting a seemingly obvious, yet critical point — every problem is different. And naturally, every problem can be placed along an axis of increasingly complexity, depending on the number of variables and scope of the task.
A simple classification I like to help frame the setup is to distinguish between open vs. closed problems:
Closed problems: contained within a system with clear and obvious boundaries e.g. a tennis match, a grade 10 math exam.
Open problems: tackled within an ambiguous, unconstrained world e.g. how to grow a business, how to implement effective economic policy.
Our education system has left us deficient in the latter. Most of us are taught only how to tackle closed problems with guided formulas and textbook examples. Yet as many young people experience, confronting open world problems outside of academia can be extremely stressful in the first years of a career (and beyond).
But as problem solvers, we must take on the responsibility to discern what’s contextually relevant. Something you can’t just assume highly-compensated consultants or your favourite Youtube celebrity may know. What's broadly required is more wisdom — but that's beyond the scope of this essay.
Problem Formulation Starts With Diagnosis & Understanding
If you want to understand the problem you’re trying to solve, you’ll need to diagnose, define and formulate it first.
I often find it useful to invert the problem I’m trying to solve first i.e. what would the opposite of good problem formulation look like? Below is what could occur within our poorly formulated problem of doubling sales:
- Boost our marketing budget
- Make critical product updates
- Improve customer retention rates
- Negotiate with manufacturers to reduce COGS
- Introduce new product lines
- Unlock new marketing channels
- Refresh our brand identity
- Reduce delivery times
- Increase prices
- Hire a bigger team to manage inbound or boost outbound sales
You see the meta problem already? Poor definitions and lack of precision in each statement, meaning solutions are nested within solutions, which in turn can nest further solutions... creating a situation with near infinite possibilities.
Take boosting the marketing budget — why should we boost the marketing budget? Where should we spend it? Which channels are most relevant? What are the alternatives? This expanse of follow-up questions come from a lack of precision. And I didn’t even flag the issue of leaping to solutions without thorough diagnosis first.
Not only is this impractical, but also inherently damaging to our mode of operating. Technically speaking, it’s known as combinatorial explosion (something I first learned via John Vervaeke’s masterful series on Awakening From the Meaning Crisis). Unbounded constraints in a problem space make any attempts at a solution utterly futile — one cannot possibly work with an infinite number of contingencies and eventualities.
Instead, we must find a way to narrow the space in which we’re operating:
- What qualitative and quantitative data do we have available?
- Is further research required? What don’t we know that we should?
- What past experiences can we rely on?
- Do we need to run some basic tests to understand what could work?
- What’s our intuition telling us?
From this, the key is to discern the relevant information to our problem.
Relevance Realisation
Relevance realisation (again, credit to John Vervaeke for inventing the phrase) boils down to this: how do we identify the most relevant information to effectively formulate our problem? Only then can we think about solving it properly.
But what does this mean in practice? Well, it’s naïve to expect a prescriptive, step-by-step formula for these types of open problems. The same principles can rarely be applied to a separate, specific context. ‘We grew our business with mass TV advertising’ won’t work for a cash-strapped startup.
And because of the lack of step-by-step guidance, it requires an integration of different modes of thinking — perspectival (the wider context) and propositional (facts we know about the problem). It’s difficult to apply an entirely rational, scientific approach to relevance realisation. What we need is an ability to discern the available information and extract the important parts from the totality of the problem space — in other words, we need insight. It’s insight that helps to piece together swathes of qualitative and quantitative data and integrate integrate it with the wisdom of experience.
This is also where we can lean on heuristics. While heuristics can have negative connotations for their overly simplistic approach to processing information, that is, paradoxically, precisely why they are useful. Without a process for contextualising, simplifying and making salient the abundance of data, our minds would (not quite literally) explode, leaving us stuck in infinite loops of thought — and combinatorial explosion once again.
The key here is to think deeply about the factors that are relevant to YOUR problem. Not necessarily blindly copying an approach you’ve heard on your favourite podcast or Twitter thread (unless, of course, it is actually relevant to the problem).
So what does attempting relevance realisation look like in practice? I like to start by taking elements surrounding the problem and organise them into questions.
💡 Can we start to make connections between elements of the problem?
- Have we seen parts of this problem before? What insights do we have?
💡 What can we categorise? (Potentially using heuristics)
- Are these elements grouped as sales/marketing, engineering or operations?
💡 What do we need to make salient?
- Are there factors which, if addressed, contribute a disproportionate amount to solving our problem?
The generalised questions can be distilled to more contextually relevant questions about the problem:
In our case of doubling sales, the following could be helpful:
Connecting the dots:
- Have we seen this problem before?
- Are there people we can speak to who have better perspective or insight into this problem?
- Are the examples of other companies in the same industry at a similar stage who’ve achieved this goal?
- Are their companies in adjacent industries with similar business models we can learn from
Understanding the specifics of our problem:
- What’s our proprietary data saying?
- What are the key insights about our customers in this circumstance that could make this context different?
- Is our company driven sales/marketing, product, virality or partnerships?
Removing what’s irrelevant:
- What can we immediately cast aside as irrelevant?
- What do we not have resources for?
- What intuitions and hypotheses map onto the data available?
- Is there anything we truly don’t know?
Cultivating awareness of pre-conceived biases:
- Are we absolutely sure of our existing beliefs?
- What are common mental models to check against? Confirmation, recency or availability bias?
The time spent investigating and scrutinizing your answers here should be positively correlated to the complexity of the problem. And there’s one thing that almost always helps at this stage: write things down. It doesn’t matter if it’s a document, spreadsheet or napkin. Writing will almost always clarifies thinking.
Secondly — use your team (if you have a team). A group’s collective intelligence often leads to swifter convergence to relevance realisation. The diversity of perspectives and experiences can plug gaps in an individual’s thinking — particularly for a general problem like ‘doubling sales’. Perhaps even more fundamentally (and I appreciate, tangentially speaking), communal exploration around problem formulation and solutions is one of the most meaningful activities humans can participate in. It’s natural to think of this in the context of sports or orchestras, but no-one really articulates this about knowledge work.
And finally, a complex problem doesn’t necessarily require a complex formulation. In fact, it can often be detrimental. You’ll often hear engineers espouse this in codebase reviews: intricately complex pieces of code may display you’re intellectual horsepower, but it’s not smart to have your team waste hours attempting to comprehend it. What’s often more intelligent is the ability to distil a problem down to its core and explain it concisely to others.
This leads us to our next step — can we agglomerate everything we’ve discussed in attempt to formulate our problem more clearly?
Returning to our original example of doubling sales in the next 12 months — we could converge on something like this:
💡 Conversion of freemium to paid customers is a bottleneck to growth — we know this because our retention rates are stable after 6 months and are in the 90th percentile for the industry and against competitors. We also have strong usage rates of the product during users’ free trials.
💡 To improve the conversion from freemium to paid, we have evidence to suggest some technical changes are required to the product, currently outside the team’s expertise — how can we put a plan in place to recruit this talent?
💡 Despite strong organic growth so far, we’ve yet to activate paid marketing. We know companies in our space have grown through paid marketing before so there’s strong precedent to prioritizing this. But we may not have the expertise.
A bad example could be something like this:
“Company Y is doing TikTok ads. We should do them too.”
- This might be true. But there’s no context about whether TikTok is the right platform for your business or whether you have the capacity to do it right now.
“Top of funnel awareness is our biggest problem”
- Again, this might be true. But is are you sure this is the problem? Does the data validate this through conversion funnels you’ve created? Is is the right time for the business to invest in awareness building?
“Let’s just make incremental gains everywhere”
- One of the most common traps for companies (particularly smaller ones) to fall into. Small gains everywhere is foolish because:
- You probably don’t have the resources available unless you’re an enormous company
- You’ll probably get better results from investing the most resources in one or two big projects
Now we’ve understood the problem, we move onto actioning it. But before we do that, I’d like to showcase one more example of outstanding problem formulation. During the height of the disastrous backlogs at the port of Long Beach a few months back, Flexport CEO Ryan Petersen provided a masterclass in problem solving.
For the sake of time, I won’t re-post the entire thread here. Instead I’ll break out the most insightful components and explain why this is such a good example of coherent problem formulation. So let’s investigate:






Now we’ve seen an expert example in action. Let’s return to forming a strategy for our problem.
Step 2: Map Onto a Strategy
Whilst a detailed breakdown of what strategy is remains beyond the scope of this essay, I’ll condense an approach here based on my preferred framework from Playing To Win by Lafley & Martin. In a business context, I believe this condenses strategy to its most fundamental components:
1. Where will we play?
- What channels and resources do we have available? This also means stating where we will not play.
2. How will we win?
- Given the above constraints, what decisions can we make to give us the best chance of success?
Back to doubling sales, we can create strategies for each of our biggest problems:
Problem #1: Conversion from freemium to paid is lower than industry benchmarks and a clear hinderance to monetization
Possible strategy:
- Address low-hanging fruit — we’ve limited the number of payment providers. Opening payment options for users to include Google Pay, Stripe and PayPal will boost conversion rates without any technical changes to the product itself
- The data scientists have conducted a linear regression suggestion the most prominent factor leading to paid conversion during the free trial is at least 5 minutes of usage in their first session — let’s build a dig into this further and prioritise crafting an experience toward hitting this metric. Let’s start by getting feedback from our most successful customers.
Problem #2: Technical engineering challenges are vital improving the product experience, but requires new expertise and a hire to the team
Possible strategy:
- Given this is such a crucial hire for the team, we need to allocate at least 30% of the founder’s time to focus on this over the next month
- Because our budgets are limited, we know we can’t offer a top rate salary for this role. Instead, we’ll need to improve our ability to ‘sell’ the company — specifically the credibility of our founding team, the quality of our product and the company vision
- Due to the technical nature of this role, engineering skills should be prioritised over people management skills. This might mean we hire up from a more junior role but with supreme technical skill.
Problem #3: We’ve yet to open up paid marketing despite clear precedent showing this is a path to boosting revenue
Possible strategy:
- Of all the performance marketing options available, Facebook ads seems the most likely to yield success — it’s the most developed ecosystem and offers the most suitable targeting options for the customers we want
- We’ll need to build out a creative asset ecosystem and pipeline given the importance of creative to the success of paid social ads
To reinforce my point, these are still hypothetical and deliberately simplistic. But as with relevance realisation and problem formulation, you want a strategy to be clear, simple and unifying — to the last note, it’s important to highlight how and why you arrived at a particularly strategy by articulating your reasoning.
Closing thoughts
It’s worth emphasising that this all sounds great in principle. But in practice, most open-world problem solving is hard. Really hard. Yet clarity of problem formulation and its subsequent solution is pivotal to the success of business success (or most all other endeavours).
My hope is this framework provides a more detailed insight into how to tackle problems independently, specifically and thoughtfully. Not merely via loosely-applied tropes like ‘being data driven’ (although as I’ve discussed, contextually relevant heuristics can be useful).
An unfortunate, yet default ‘we-can-fix-it-all’ approach is a recipe for burnout and underperformance. But focused teams can make extraordinary progress. And an underappreciated topic is the meaning people derive from their work — usually from progressing as a group of talented and competent operators toward a deliberate and well-defined goal.
With sufficient cycles of problem-solving comes attunement to the nuances of the space you’re operating in — which compounds into a self-propagating feedback mechanism over time, constantly accelerated the speed of learning. This is perhaps the most important aspect of the whole process: we can improve our ability to reflect, self-correct and examine to become better problem solvers. This higher attunement continuously supports more relevant insights.
As always, please feel free to get in touch to highlight any gaps in my thinking — there are likely many things I’ve yet to comprehend. I always find it helpful to appreciate that wisdom is a process, not a destination.
References
- Awakening From The Meaning Crisis by John Vervaeke (episodes 26-32)
- How To Solve It — G. Polya
- Playing To Win, How Strategy Really Works — A.G. Lafley & Roger Martin
- How Brands Grow — Byron Sharp
- Practical Wisdom — Barry Schwartz and Kenneth Evan Sharpe
- Boyd: The Fighter Pilot Who Changed The Art Of War — Robert Coram